News Item
On June 8, 2023, Tobin Center Associate Faculty Director Zack Cooper testified before the US Senate Committee on Finance, serving as a key expert for the hearing “Consolidation and Corporate Ownership in Health Care: Trends and Impacts on Access, Quality, …
Relevance: 2.9239612
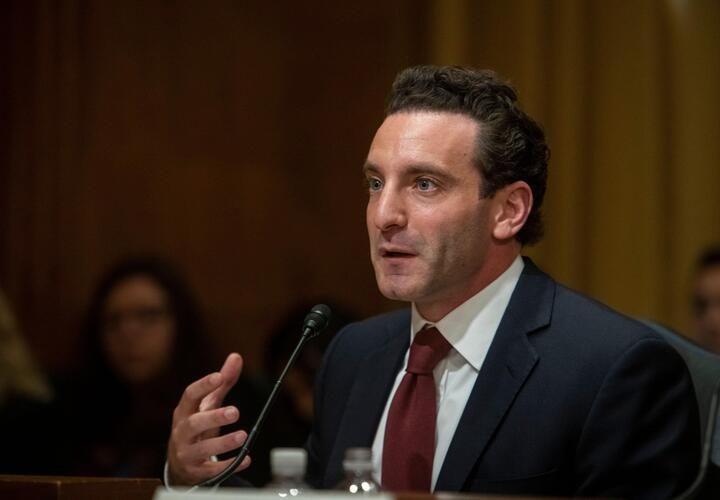